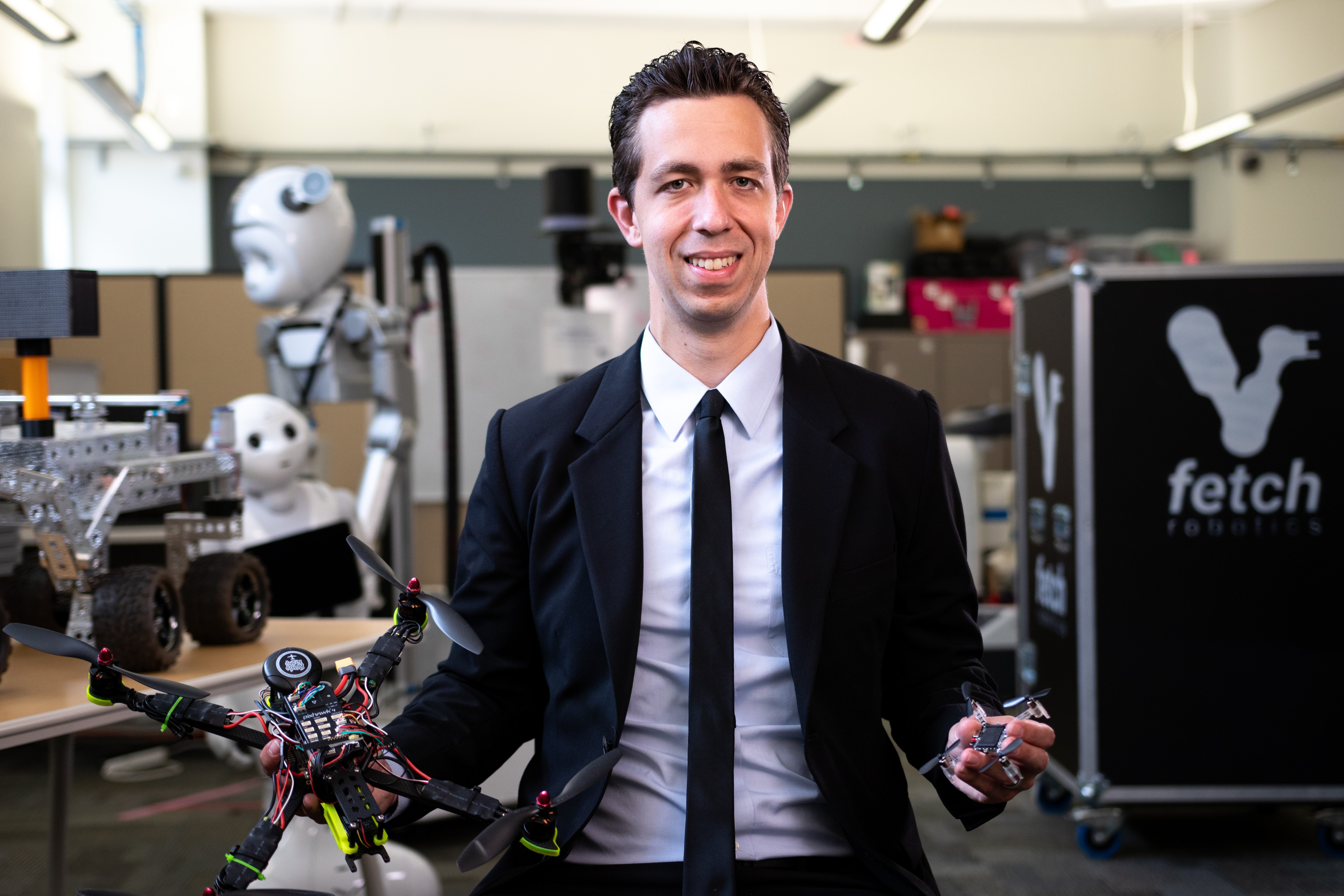
Georgia Institute of Technology
I work to democratize robotic technology – placing the power of robots in the hands of everyone by developing new computational methods and human factors insights that enable robots to 1) learn novel skills via human demonstration from diverse end-users, 2) understand and support the human in human-robot interaction (HRI) including through explainable Artificial Intelligence (xAI), and 3) coordinate human-robot teams. I employ human-subjects research methods to inform the formulation of our systems, validate our methods improve HRI across both subjective and objective metrics, and develop design guidelines for researchers and practitioners alike.
My research is inspired by the potential of robots to become an invaluable part of our everyday lives, from autonomous vehicles (AVs) to healthcare, manufacturing, and defense. To reach this potential, however, we must move away from army-of-consultants approaches that yield expensive, ad hoc, solutions and move towards interactive learning techniques, e.g. Learning from Demonstration (LfD), in which robots learn skills (e.g., an AV navigating an obstacle course with user-specific objectives) via human feedback (e.g., robots observing human skill performance). Likewise, robots must support humans through human-centered design techniques, explainable Artificial Intelligence (XAI), and team coordination algorithms.
My lab, the CORE Robotics Lab, is supporting the AI-CARING institute by developing safe and multi-model robot programming by demonstration techniques that could be used by care partners (e.g., spouse, adult children, nurses) to program robots to assist in daily activities of living. We are actively researchers mechanisms to integrate Multi-modal Large Language Models (LLMs) with Interactive Machine Learning (e.g., Inverse Reinforcement Learning) to enable humans to intuitively program robots to perform assistive tasks in the home (e.g., cooking and cleaning). We design our models to be personalizeable to capture user-specific preferences for how the robot should behave and encode social norms. We employ mixed-methods research with our target population of care members and partners to inform the design of and evaluate our technical contributions. Conducting focus groups, prototyping activities, and feedback sessions are vital research activities that our lab benefits from in meeting the needs of our target population.
The proposed outcome of our research is a set of robotic algorithms and interfaces that help realize our vision for assistive robots in the home to help older adults age more gracefully, staying in the home and in their communities, and avoid costly care in an assistive living environment.