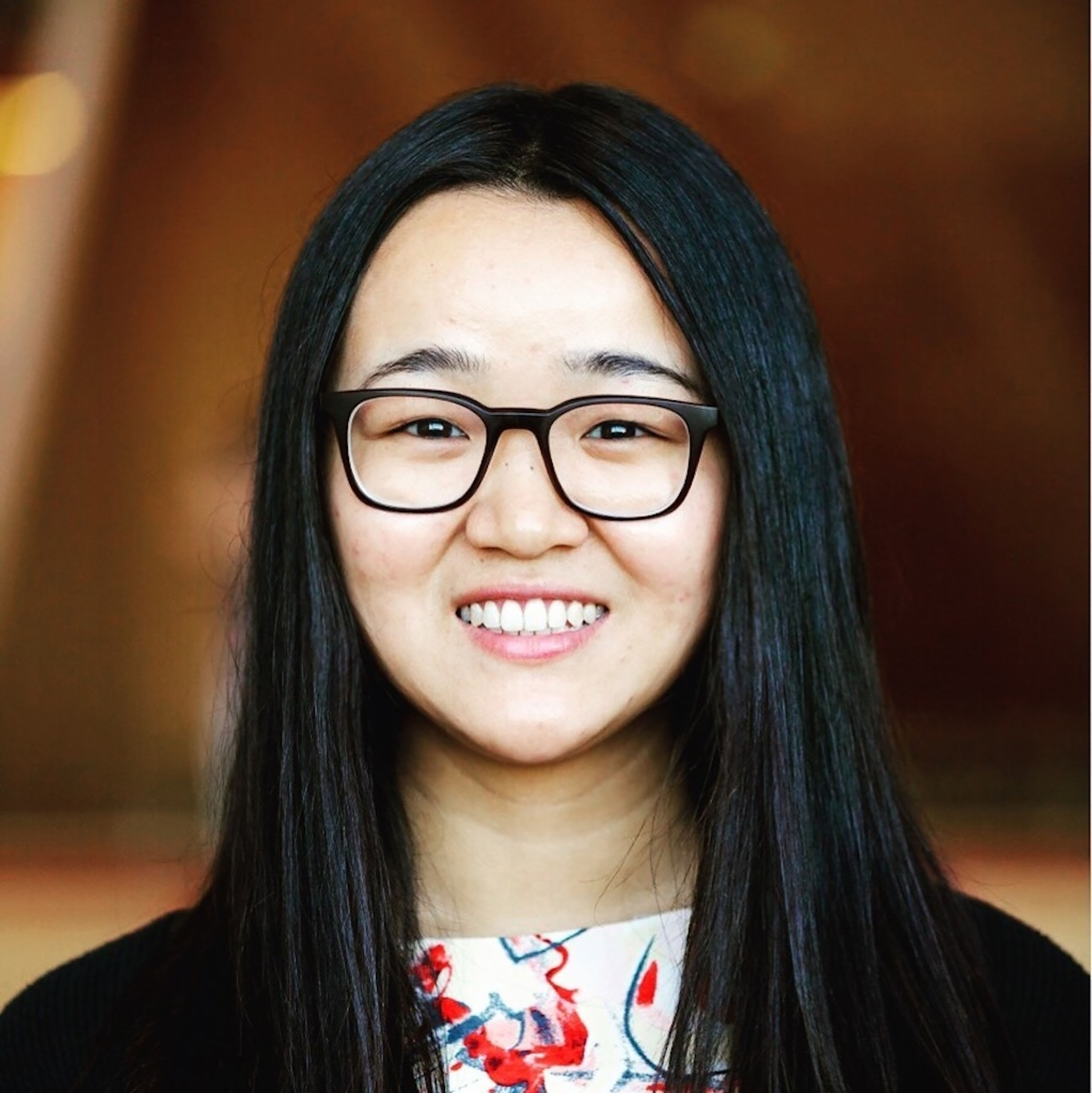
Stanford University
My research goal is to better understand human communication in social context and build socially aware language technologies to support human-human and human-computer interaction. Related to AI-CARING, I am excited to build interactive LLM-empowered feedback systems to help caregivers learn support provision skills. Generative models like GPT3 perform really well out of the box but are not suited for empathic conversations especially for the specific MCI context with caregiving and social support exchange. Through our project, we aim at building a training conversational agent to help caregivers frame supportive, reflective, and more helpful responses to support seekers. More importantly, we are interested in deploying this type of system with caregivers to demonstrate the effectiveness of our training system, and understand its broad implication in eventually supporting MCI members.
As an initial effort towards this goal, we currently look at online peer counseling platforms to see how we can build such tools to help counselors. Peer counselors are key to the success of online peer counseling platforms, but most of them often do not have systematic ways to receive guidelines or supervision. We have developed the first version of a system called CARE: an interactive AI-based tool to empower peer counselors through automatic suggestion generation. During the practical training stage, CARE helps diagnose which specific counseling strategies are most suitable in the given context and provides tailored example responses as suggestions. Counselors can choose to select, modify, or ignore any suggestion before replying to the support seeker. Building upon the Motivational Interviewing framework, CARE utilizes large-scale counseling conversation data together with advanced natural language generation techniques to achieve these functionalities. We demonstrate the efficacy of CARE by performing both quantitative evaluations and qualitative user studies through simulated chats and semi-structured interviews. We also find that CARE especially helps novice counselors respond better in challenging situations. We're in the process of conducting large-scale evaluations to see how CARE can help caregivers in general.
This work holds great potential in facilitating more effective verbal communication between caregivers (support providers) and MCI members (support seekers). It would help support older adults especially in terms of NLP-based nudging techniques.