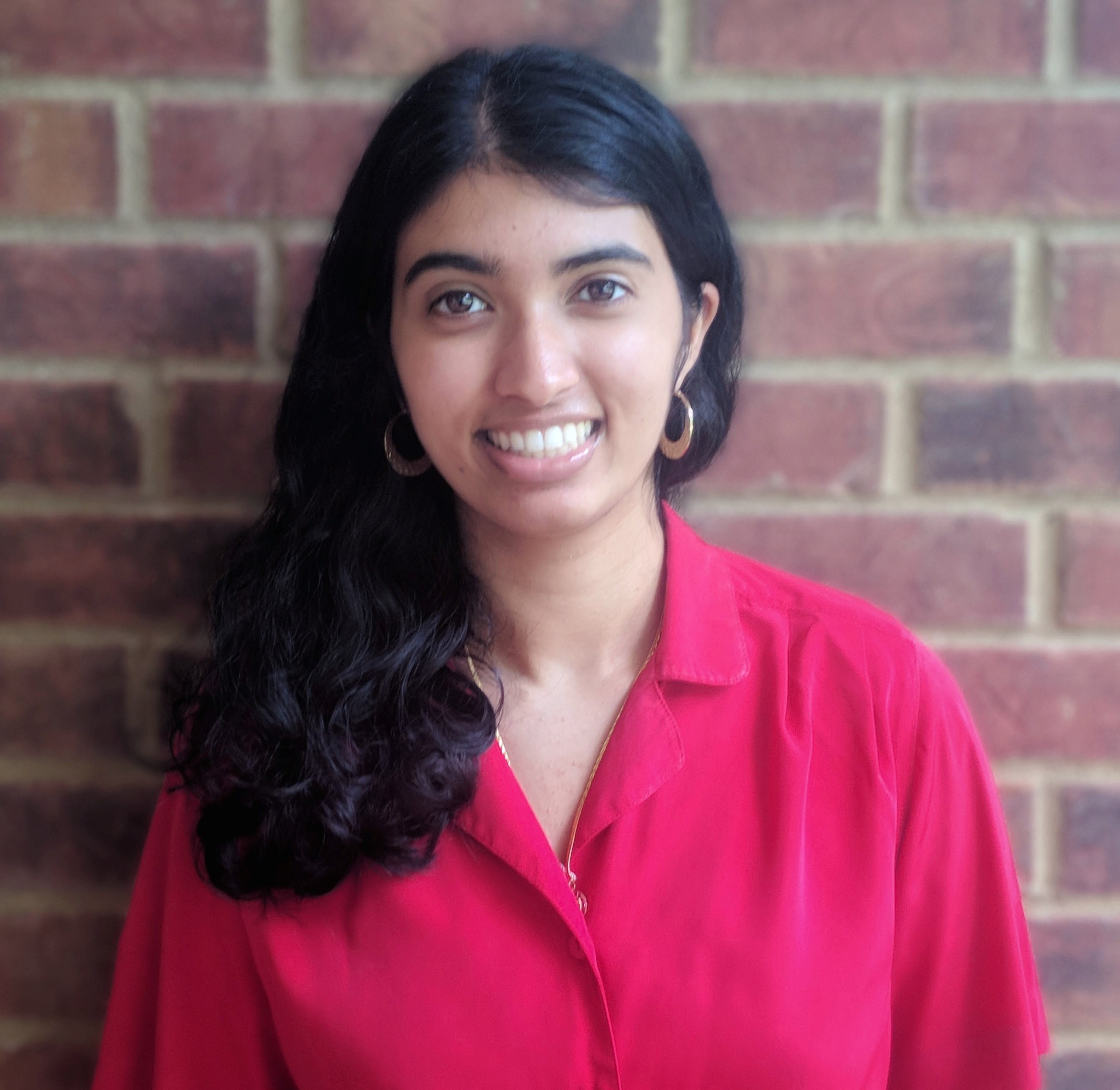
Carnegie Mellon University
Different people respond to feedback and guidance in different ways. Some might prefer a firmer approach, some might prefer softer. Their preferences may even change depending on their mood, the time of day, physical health, etc. My researcg aims to learn people’s preferences for both verbal and non-verbal feedback and guidance by observing their reactions to different types of feedback as they perform various physical activities in various contexts (e.g., time of day, physical and emotional state, etc.). We plan to treat this as a contextual multi-armed bandit problem, where observing the outcome of the feedback (e.g., does the person do what has been suggested, does their affect change in a positive or negative way) provides a (weak) signal to the AI agent. We will use mainly non-verbal cues from people – facial expressions, posture, gestures – to infer their mental state and the AI agent will respond, in kind, with facial expressions, posture, gestures, and verbal feedback and guidance. In previous studies in the educational domain, we saw a significant increase in learning when the robot exhibited an emotional response to the user’s actions, as opposed to a neutral robot that just provided verbal feedback. Other works have shown that tailoring the feedback to the person’s personality is effective. We believe that a similar approach, but one that learns from observing how people react to the feedback, will have even greater utility.
Related Publications:
[Conference] R. Kaushik and R. Simmons, "Affective Robot Behavior Improves Learning in a Sorting Game," in the International Conference on Robot & Human Interactive Communication 2022
[Workshop] R. Kaushik and R. Simmons, "Context-dependent Personalized Robot Feedback to Improve Learning," in the Context-awareness in HRI Workshop (part of the Human-Robot Interactions Conference 2022)
[Conference] R. Kaushik and R. Simmons, "Perception of Emotion in Torso and Arm Movements on Humanoid Robot Quori," in Companion of the 2021 ACM/IEEE International Conference on Human-Robot Interaction, 2021.
[Conference] R. Kaushik and R. Simmons,"Early Prediction of Student Engagement-related Events from Facial and Contextual Features" in the International Conference on Social Robotics, 2021.