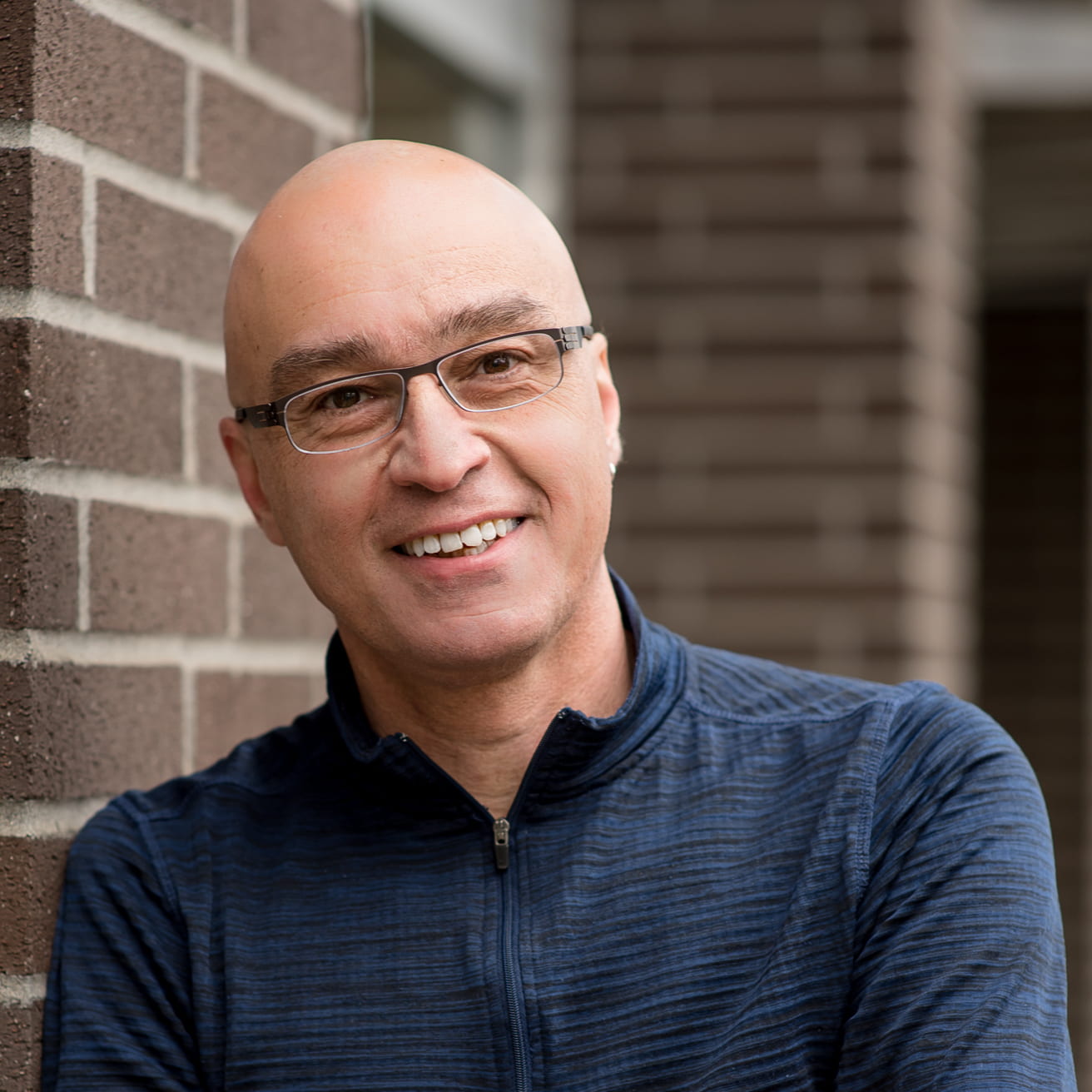
Oregon State University
Kagan Tumer’s research focuses on learning and coordination in complex domains with an emphasis on multiagent learning and learning in domains where the task cannot be adequately captured with a single reward function. His research addresses two simple questions: (i) how to ensure that what’s good for an agent is good for the system; and (ii) in long-term tasks, how to determine what matters when?
To address the first question, Kagan’s work derives reward functions that “translate” the system objective into agent objectives. Mathematically, the “difference reward” quantifies an agent’s contribution to the system goals by isolating its marginal impact. To address the second question, Kagan’s work derives population dynamics that coevolves agents by selecting them both for individually optimizing specific objectives and for how well they address a system’s (possible conflicting) long-term system goals.